Abstract
Does network recruitment contribute to the glass ceiling? We use administrative data from two companies to answer the question. In the presence of gender homophily, recruitment through employee referrals can disadvantage women when an old boys’ network is in place. We calculate the segregating effects of network recruitment across multiple job levels in the two firms. If network recruitment is a factor, the segregating impact should disadvantage women more at higher levels. We find this pattern, but also find that network recruitment is a desegregating force overall. It promotes women’s representation strongly at all levels, but less so at higher levels. This article shows how administrative data can be used to tackle the complex problem of gender inequality in organizations to counter the glass ceiling.
Many studies have adopted the glass ceiling metaphor to describe the phenomenon where gender inequality in outcomes is more severe at the top of the reward distribution. Although many other factors are theorized to be at play, some recent research has sought to understand the external sources of the glass ceiling pattern (Gorman and Kmec 2009; Fernandez and Abraham 2010, 2011). One factor that is often invoked to account for gender differences in outcomes is firms’ reliance on hiring through social networks. Numerous studies have documented the segregated nature of social networks (see, for example, Brass 1985; Campbell 1988; Ibarra 1992; Lincoln and Miller 1979; Marsden 1987, 1988; Moore 1990; Straits 1996). This line of reasoning states that in the presence of gender homophily, recruitment through employee referrals is likely to further disadvantage women in hiring. This logic is often connected to the glass ceiling by arguing that recruitment to higher levels of the organization is likely dominated by an old boys’ network. Even in the absence of gender biases, homophily among successful men can effectively restrict access to the upper echelons of an organization in a manner that obstructs women’s success. The logic of this argument is clear. But is there empirical evidence for its presence and operation?
In this article, we look for evidence for whether network recruitment contributes to a glass ceiling phenomenon using administrative data from two distinct organizations. Although politically quite sensitive, the data required for this exercise is commonly available at most mid- to large-size organizations. Prior scholarship has documented a method for measuring the segregating effects of network recruitment (Rubineau and Fernandez 2015). Organizations with referral bonuses commonly collect the application, hiring, and referring data needed to use this method. The authors have worked in collaboration with two large pharmaceutical organizations to identify and collect relevant data to help these firms in pursuit of their gender equity goals. We leverage their existing human resources (HR) data to measure the segregating effects of network recruitment. Specifically, we estimate these segregating effects at multiple organizational levels in each of the two companies. We examine whether these estimated segregating effects are consistent with the predicted glass ceiling pattern and discuss the practical and theoretical implications of our findings.
We first bring empirical evidence to the prior assertion-based arguments regarding a possible role of network processes in contributing to a glass ceiling effect. In doing so, we demonstrate how existing private-sector organizational HR data resources can be leveraged to understand and manage the segregating effects of network recruitment. The article thus serves as a powerful example of how administrative data—the residuum of modern bureaucratic processes (Reardon 2019)—can be harvested and put to use in addressing important science and policy questions.
Both firms studied yield the same paradoxical results. First, we find evidence of a clear glass ceiling pattern: network recruitment produces a decreasing percentage of women as one looks across levels within the firms’ hierarchies. Second, we find that network recruitment tends to act as a desegregating force. That is, network recruitment tends to push firms to become more female than they would be without network recruitment. Although network recruitment is a desegregating force overall, it promotes women’s representation more strongly at lower levels within the firms, and less strongly at higher levels.
THE GLASS CEILING AND NETWORK RECRUITMENT
The term glass ceiling has become a general metaphor applied to the topic of gender inequality in the workplace and in organizational governance (Hymowitz and Schellhardt 1986). Put simply, the glass ceiling is meant to imply that women encounter invisible barriers when attempting to move up corporate hierarchies. Women may be able to enter the workforce with little resistance, and even attain near- or beyond-parity representation in lower-level jobs. But when a glass ceiling is in place, women seeking positions of leadership, power, and status in their organizations face a gendered obstacle. Although coined more than thirty years ago, the metaphor has retained its currency in the face of women’s enduring relative absence in high positions in corporations (Cotter et al. 2001; Glass and Cook 2016; Tinsley et al. 2017; Yap and Konrad 2009).
The Glass Ceiling and the Labor Market
Much scholarship has sought to identify the mechanisms that produce this gendered pattern of hierarchical advantage (see Petersen and Saporta 2004). Although many glass ceiling studies invoke the image of an internal labor market or a within-firm job ladder, recent work argues that the pattern applies to the labor market more broadly (Fernandez and Campero 2017; Fernandez-Mateo and Fernandez 2016). In this work, the glass ceiling is seen as a vertical form of more general gendered job segregation patterns (Fernandez and Campero 2017; Fernandez and Abraham 2010, 2011; Fernandez-Mateo 2009). In this respect, insight into the phenomenon can be gained by drawing on the rich research addressing job sex segregation (for example, Bielby and Baron 1986; Jacobs 1989). Some of these mechanisms are posited to operate on the demand side of the labor market (Cejka and Eagly 1999; Kmec 2005). Others argue that supply-side processes are also likely to contribute to job sex segregation (Correll 2001; Fernandez and Friedrich 2011; Barbulescu and Bidwell 2013; Fernandez and Campero 2017).
Whatever the sources of this segregation, addressing the enduring inequality in gendered organizational attainment requires revealing and resolving the mechanisms that generate it (Reskin 2003). Complicating the task are the many mechanisms interacting simultaneously and working toward preserving the inequality (Ridgeway 1997). This article seeks to contribute to the scholarly understanding of the glass ceiling phenomenon by making a detailed empirical examination of one commonly discussed mechanism seen as contributing to the glass ceiling—network recruitment.
Network Recruitment and the Old Boys’ Network
One of the factors often invoked to account for gender differences in labor market outcomes is firms’ reliance on hiring through social networks. Network recruitment continues to be both a dominant and an effective recruitment method (Breaugh 2014; Marsden and Gorman 2001). In relying on social networks, however, employers inherit the biases within them. Numerous studies have documented the segregated nature of social networks generally (Brass 1985; Campbell 1988; Ibarra 1992; Lincoln and Miller 1979; Marsden 1987, 1988; Moore 1990; Straits 1996). Just as many have documented the gender segregation of workplace networks specifically (Belle, Smith-Doerr, and O’Brien 2014; Fernandez and Sosa 2005; Kleinbaum, Stuart, and Tushman 2013; Lawrence 2006). Because of gender homophily in workers’ networks—the tendency for networks to be same-sex—network recruitment has been argued to disadvantage women in hiring (Acker 2009; Beaman, Keleher, and Magruder 2018; Leicht and Marx 1997; McDonald 2011; Reskin, McBrier, and Kmec 1999).
The expected gender-segregating effects of network recruitment sometimes explicitly invokes the old boys’ network (see, for example, McDonald 2011, 317). Despite the strong conventional wisdom that network recruitment would be expected to have segregating effects, empirical studies seeking to evaluate these effects have sometimes yielded contrary findings. For example, a study of hospitals in the northwestern United States finds that organizations that engaged in higher levels of network recruitment exhibited lower levels of gender segregation (Kmec 2008). In another example, a big-data study examining the segregating effects of network recruitment in Stockholm finds that increased use of network recruitment was associated with a decrease in the level of gender segregation in the labor market (Collet, Hedström, and Johansson 2014). These and a variety of other empirical findings challenge the idea that network recruitment necessarily perpetuates or exacerbates segregation (see Rubineau and Fernandez 2015).
To better understand the segregating effects of network recruitment, we developed a line of research-building theory regarding the dynamics relating network recruitment to segregation. This effort yielded two articles examining how network recruitment affects hiring outcomes (Rubineau and Fernandez 2013, 2015). The articles show, first, that referrers play an important role in determining the segregating effects of network recruitment (2013), and, second, that measures taken from HR data involving job applications and referring ties can reveal the equilibrium composition toward which network recruitment will push a job’s gender composition (2015). This equilibrium indicates the segregating effects of network recruitment in isolation—network recruitment’s push toward or pull from segregation. The key determinants are not the initial composition of the job or firm but instead the gender differences in two referring-related rates: one at which employees refer generally and another at which referring employees specifically refer same-sex contacts to apply to the firm. In the presence of homophilous referring, if men and women differ in their rates of producing referral applicants, then, ceteris paribus, the equilibrium gender composition from referring will favor the group that produces more referral applicants. Similarly, if the men and women who refer differ in their rates of referring same-sex contacts, then the equilibrium gender composition from network recruitment will favor the group that generates a higher proportion of same-sex referral applicants.
In addition to providing analytical bite on the general issue of network recruitment, these articles yield important practical implications for firms seeking to affect the gender compositions of their workforce. The method requires that the firm follow formalized HR practices and data recording protocols for both the hiring process, beginning with job applications, and the referral bonus administration that links current employee referrers with their referral applicants (Rubineau and Fernandez 2015). Although rarely systematically analyzed, most mid-sized to large firms routinely track the data required to shed light on how network recruitment is affecting their gender composition.1 Given enough observations, the segregating effects of network recruitment could be calculated not only on a firm level, but also subdivided into useful subsets. In this article, we look at the segregating effects of network recruitment for lower-level jobs within the firm relative to those for higher-level jobs to examine whether and to what extent network recruitment may contribute to a glass ceiling effect. By applying this method, we can test for evidence that network recruitment contributes to the glass ceiling phenomenon.
Specifying Expectations: A Glass Ceiling Pattern
If network recruitment does contribute to a glass ceiling effect in organizations, how would the contribution be manifest and how could it be documented? The method just described enables the calculation of the segregating effects of network recruitment in terms of the equilibrium composition of women toward which network recruitment pushes the current incumbent composition.
If a glass ceiling effect derives in part from network recruitment dynamics, then we would expect a specific pattern in the relative calculated equilibrium compositions of women produced by network recruitment at different ranks within a firm. More specifically, the equilibrium composition of women produced by network recruitment operating at higher levels of the firm would be expected to be lower than that at lower levels of the firm. By measuring the segregating effects of network recruitment for multiple job levels within a single firm, we can evaluate whether network recruitment dynamics contribute the expected glass ceiling pattern.
We undertake this analysis for two organizations and in doing so attempt an empirical existence proof. Evidence that network recruitment yields the expected glass ceiling pattern would provide empirical support allowing us to rule-in the claim that this is one of the many mechanisms contributing to a glass ceiling. Absence of such evidence could not be taken as evidence that this mechanism never contributes to a glass ceiling. Instead, such absence of evidence would indicate that although network recruitment could potentially contribute to a glass ceiling in other contexts, the current empirical contexts would not provide unambiguous evidence for such a contributory role. Although recognizing the logical status of the evidence presented here is important for scientific progress in this domain, it is also important to understand that from the perspective of the cooperating firms, these analyses are inherently of interest for furthering their own understanding of how their policies around network recruitment contribute—or not—in their pursuit of gender equity in their organizations.
DATA AND METHODS
We approached the companies studied here through professional connections developed in the course of our teaching business classes at our respective universities. In both cases, the contacts suggested that their companies were interested in deeper understanding of issues of gender diversity. After a period of discussion with the firms’ management, we presented a research plan and developed a mutually acceptable confidentiality and nondisclosure agreement. We then worked with company personnel to identify the relevant databases and to match and merge these various sources using anonymized data.
The estimation of the segregating effects of network recruitment requires a specific set of HR data regarding organizational recruitment and referring. These data requirements are summarized here (for a full description, see Rubineau and Fernandez 2015). As numerous other articles in this volume demonstrate, when dealing with administrative records, multiple data sources often need to be matched and combined to triangulate on the information of interest (for example, Penner et al. 2019). This is true in our case as well. The required data for these analyses are coming from several disparate databases that tend to be maintained by different organizational subunits, and in our private-sector cases, these subunits are housed under the umbrella of the HR department of these organizations.2
First, to characterize the hiring pipeline into the firm, the method requires data on all job candidates’ gender, not simply the gender of the people hired (for a discussion of “start with hire” analyses, see Fernandez and Weinberg 1997). This is because relative to post-hire data, the pre-hire pool more accurately reflects the supply-side biases affecting nonreferral applicants’ decisions to apply for a defined job, as well as the referring behavior of employee referrers.
Second, we need to know the referral status for each candidate. This information is typically included in a database produced by applicant tracking software managed by the recruitment arm of the human resources department. However, for each of the referral candidates, we also need to know the identity of their corresponding employee referrer so as to identify the referrer’s gender and level in the organization. In our cases, information on referrers is generated by an HR team that administers the firms’ employee referral bonus programs.
In addition to data on the hiring pipeline, the method also requires data for current employees and employee referrers. Specifically, we need to know all employees’ gender. These are contained in the companies’ human resources information system (HRIS). We combine the HRIS data with information from the employee referral bonus program to identify whether employees have attempted to refer someone; for those who have, we identify their corresponding referral applicants in the applicant tracking system.
To provide better understanding of the gender patterns across the organizational hierarchy, this analysis also requires grouping these data elements by job level within the firm. For these reasons, we also need to identify the job levels of the job opportunity for which applicants apply, and the job levels of all current employees. These too are taken from the companies’ HRIS databases.
As described, we identified two organizations (Firm 1 and Firm 2) willing to cooperate and provide the needed data elements. Both are large U.S. pharmaceutical industry firms and provided data on their job recruitment efforts and referral bonus program data for the 2014 calendar year. The choice of these firms was made based primarily on our ability to establish data-sharing and collaborative research relationships with the firms. We neither claim that pharmaceutical firms are particularly likely or unlikely to exhibit a glass ceiling phenomenon, nor would such a claim be required for conducting the current analysis.
The jobs in each of these two organizations are divided into four groups of job levels. Contacts at Firm 1 provided the needed data already grouped by job-level groups based on their understanding of meaningful division lines among job levels. Firm 2 provided detailed job-level data, and the researchers divided these observations into four groups based on a combination of job categories (for example, nonsalaried nonexempt jobs, hourly, and unionized jobs, as level 1, and salaried exempt jobs as progressively higher levels), and the sizes of the specific job levels to allow for approximately similarly sized job level groups. The job-level groupings for the Firm 2 data were based on the job level of the referrer alone, as the job applicant data did not allow for clear matching of job levels. We discuss the implications of this assumption later. For Firm 2, the gender of job applicants was coded probabilistically by comparing applicants’ first names against a database of first names used by people in the United States and their probability of being associated with men and women as provided by Genderize.io.3 Table 1 provides a summary of the nature of the recruiting and referring processes for these two organizations.
HR Recruitment and Referring Data
RESULTS
The variables estimated from the two datasets are described as follows (letter designations from Rubineau and Fernandez 2015). Their estimates for the four job levels in the two organizations are presented in table 2.
Measures and Calculations for Equilibrium Gender Composition from Network Recruiting
(A) Percent male among applicants referred by men
(B) Percent female among applicants referred by women
(C) Percent referrers among men at firm
(D) Percent referrers among women at firm
(f) Percent female of nonreferral applicants
(s) Supply-side effects parameter = f/(1 – f)
(d) Demand-side effects parameter = D/C
(m) Men’s same-sex referring rate = As/(1 – A + As)
(w) Women’s same-sex referring rate = B/(s – Bs + B)
The first five variables are estimated from the data provided by the two firms, and the next four terms are calculated using the previous five. These last four terms (s, d, m, w) are entered into the following equation (Rubineau and Fernandez 2015, 1654). The equation gives the equilibrium percent female toward which network recruitment is pushing the composition of incumbents.
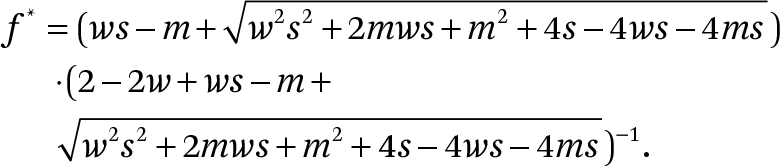
The last column of table 2 shows the aggregated results (without respect to organizational level) for the two firms as a whole. Overall, both firms are near gender parity: females in Firm 1 are 52 percent of incumbent employees; Firm 2 is 47 percent female. Interestingly, by itself, network recruitment dynamics are pushing both firms to be even more female than they already are. For Firm 1, the equilibrium percent female is 55 percent (versus 52 percent of incumbents), and at Firm 2 the equilibrium is 51 percent (47 percent of incumbents).
Equilibriums Across Job Levels
We next look at how these dynamics play out at each level of these organizations. The columns of table 2 provide the results of these calculations along with the gender composition of incumbents in each of the four job levels in the two firms. For Firm 2, the f term—percent female among nonreferral applicants—was not available separately for each of the job levels. This percentage was available only for the entire applicant pool (39 percent). Because of this limitation, as a type of sensitivity check, we calculated two sets of equilibriums for the four job levels of Firm 2. One set used the 39 percent value for all four job levels as the f term. A second set used the current composition of incumbents. These two sets of calculations yielded almost identical values, showing that the sensitivity of the equilibriums to the value of this variable is relatively low.
Table 2 shows that for both Firm 1 and Firm 2, we find the same paradoxical result. First, we do find evidence of a strikingly clear glass ceiling pattern: the effects of network recruitment are a decreasing percentage of women with increasing levels within the firms’ hierarchies. In Firm 1, network recruitment pushes the lowest-level jobs toward a 60 percent female composition, but the highest-level jobs toward a below-parity 48 percent female composition. The two job levels between these ends also have intermediate equilibrium compositions, resulting in a monotonic decline in the gender integration effects of network recruitment. This monotonic decline is also illustrated in the solid line in figure 1.
Comparing Equilibrium Gender Compositions Produced by Network Recruiting
In Firm 2, we see an almost identical pattern. Network recruitment pushes the lowest-level jobs toward a 55 percent female composition, but the highest-level jobs toward a below-parity 47 percent. The trend across the four job levels in Firm 2 is also a monotonic decline, illustrated graphically in the dashed line in figure 1. Results from both firms examined are consistent in exhibiting the pattern of results that would be expected if network recruitment contributed to a glass ceiling effect.
These glass-ceiling-consistent results do not mean, however, that network recruitment is acting as a segregating force at every level of the two firms. By comparing the calculated equilibrium compositions with the composition of incumbents, it is clear that network recruitment tends to act as an integrating force even within each level. As shown in the final rows of the two panels of table 2, for all job levels except level 3 (coincidentally for both firms), incumbents are less female than the equilibrium entailed by network recruitment. This result means that network recruitment acts to make these jobs more female than they would be otherwise. This integrating effect of network recruitment is strongest for the lowest job levels and weaker for the higher job levels. In this way, we have network recruitment exhibiting the curious behavior of simultaneously contributing to the gender integration of the firms but also contributing to the glass ceiling patterns in both firms.
Segregating Effects of Network Recruitment
The equilibriums in table 2 illustrate not the projected future composition of each job level for each firm, but instead the conceptual target composition toward which network recruitment—in isolation from other composition-altering mechanisms—is pushing those jobs. That is, if the HR processes in the firms could reach a state at which the only mechanisms affecting the compositions of the job levels were the rate and gender composition of nonreferral applicants, network recruitment, and otherwise gender-unbiased hiring rate and exit rates, then the equilibriums would be the projected future composition of each job level. This hypothetical state is not an actual goal but a useful conceptual baseline to illustrate the segregating effects of network recruitment.
The calculated equilibriums themselves are not the sole indicator of the segregating effects of network recruitment. If network recruitment were pushing two sets of jobs both toward a 60 percent female equilibrium composition, and the first set had an input composition of 30 percent female, and the second set a composition of 55 percent, we would know that the network recruitment is exerting a larger push on the composition of the first set of jobs than on the second set. The direction and magnitude of the difference between the input composition and the calculated equilibrium composition from network recruitment serves as one way to quantify the composition-changing effects of network recruitment.4 Table 2 provides these percentage-point difference measures for the four job levels and the overall firm for both firms.
We also provide a second, and possibly more easily interpreted, measure of the composition-changing effects of network recruitment: the magnitude of another well-understood segregating mechanism—an explicit bias in screening applicants—that would be needed to yield the equivalent compositional changes as produced by network recruitment (first described in Rubineau and Fernandez 2013). Using the examples from the previous paragraph, how much of an explicit preference for hiring women over men would be needed to move one set of jobs with a steady input composition—30 percent female—to yield a final stable job composition that is 60 percent female, and how much of an explicit preference would be needed to move another set of jobs from 55 percent female to 60 percent?
We provide this answer by solving a probability problem. If the entering composition of applicants is p (in this example, 30 percent female or 55 percent), what odds for choosing a female for hire are needed to yield the target equilibrium composition (in this example, 60 percent female)? When the odds are 1, then the equilibrium composition necessarily equals the input composition. Greater than 1 odds for choosing a female for hire will push the composition more female than the input composition, and below 1 odds for choosing a female for hire will push the composition more male. The two odds in the example are 1.11, and 1.87, respectively.
We solve this problem for the four sets of job levels as well as for all jobs in the two firms. The calculated odds shown in table 2 represent the odds for choosing a female for hire necessary to push the input compositions (measures f in the table) to the equilibrium compositions from network recruitment (indicated in the table in bold). This odds measure is a useful summary metric for the strength of the segregating effects of network recruitment. The magnitudes of the odds shown in table 2, ranging from 0.92 to 1.40, are substantial. These substantial pushes to the composition of jobs in the two firms illustrate the power of network recruitment to alter the gender compositions of jobs in firms. This power can also be wielded as a tool for achieving organizational diversity goals. Our analysis of these administrative hiring and referring data provide additional insights regarding the likely effectiveness of using referring to achieve organizational diversity goals.5
Effects of Different Referring Behaviors
Additional insights can be gleaned from close scrutiny of the terms in table 2 for the two organizations. With respect to policy choices, which can affect the equilibrium toward which network recruitment is pushing the firm, two basic levers are available. First, firms can seek to encourage more referring from the underrepresented group relative to the overrepresented group. In terms of the parameters, this implies that D (the referring rate for females) should be made greater than C (the referring rate for males). Second, firms can encourage referrers to target the underrepresented group in their referring.6 In these cases, this corresponds to having males produce relatively more female referrals (a low level of A), and have females produce a high level of female referrals (a high level of B).
Comparisons between Firm 1 and Firm 2 at low and high levels help reveal the relative strength of these levers. At the lowest level of both firms, the first lever is clearly active: D is substantially greater than C. This contributes to pushing the equilibrium composition of females at the lowest level to be substantially higher than the current percent female among incumbents. In contrast, at higher organizational levels, the gap between D and C is much smaller, and thus cannot serve to differentiate these two cases.
At the high end of Firm 1—but not Firm 2—we see evidence of the second lever at work: the majority of referrals produced by males are female (A is < 0.5), and the majority of referrals produced by females, are also female (B is > 0.5). Although the contrast between the two firms in these rates is striking, the resulting equilibriums are not at all different across the two firms, and not as high as the equilibriums for the lower-level jobs. From the percentage-point change from input to equilibrium, and from the equivalent odds measure of the strength of the compositional push from network recruitment, it is clear that this second lever can produce substantial changes. These changes, however, cannot overcome the very low composition of the inputs, and the net effect remains that higher-level jobs are pushed toward a composition that is less female than lower-level jobs. This suggests that at least in these cases, lever 1—getting the underrepresented group to refer more—may be the more reliably beneficial approach when using network recruitment to ameliorate the glass ceiling.
DISCUSSION AND IMPLICATIONS
Although network recruitment processes have often been invoked as a contributor to the glass ceiling phenomenon, little empirical evidence has been presented to demonstrate such an association. Prior to this project, the relationship has largely been putative. This article leverages detailed HR data regarding recruitment and referring processes in two firms to empirically evaluate whether network recruitment contributes to a glass ceiling effect in the two firms.
This evaluation process first calculates the segregating effects of network recruitment for the firm overall. We then divide the data from each firm into four job-level categories, representing increasingly elite echelons within each firm. If network recruitment were to contribute to a glass ceiling phenomenon, then the calculated effects of network recruitment would be a declining gender composition at higher job levels within the firm. We find precisely and unambiguously this pattern in both firms.
Grouping the Data by Job Level: Approaches and Implications
Grouping data from the two firms by job level was accomplished in potentially different ways. Job referrers are not necessarily at the same job level as that of the job opportunity to which they refer. Grouping firm referring data into job levels is done in one of three ways. One approach is to limit referrers and referral applicants who are referring from and applying to the same job level. A second approach is to define the job level by that of the referrer. A third approach is to define the job level by that of the job to which the referral applicant applies. Firm 1 calculated the parameters we requested, but we do not know for certain which of the three approaches was used. For Firm 2, we necessarily determined job level by the job level of the referrer—the second approach. We examine the implications of these approaches.
Might the job grouping approach affect the resulting pattern we observe? Although we cannot test the assumption using our data directly, we consider its possible implications. First, extensive scholarship shows that people tend to refer homophilously, including on characteristics such as educational background, professional behavior, job-related aptitude, and corporate division (Fernandez, Castilla, and Moore 2000; Rider 2012; Burks et al. 2015; Hensvik and Skans 2016; Brown, Setren, and Topa 2016). Also, our job-level categories are broad. For these reasons, we do expect the cases where referrer and applicant are at the same broad job-level category to have the most observations.
Second, both theoretical and empirical network recruitment scholarship supports the idea that referrers tend to refer for job opportunities at their own level or lower more than for job opportunities at a higher level than the referrer (Leicht and Marx 1997; Brown, Setren, and Topa 2016). Building on this finding, we examine the likely effects of the grouping-by-referrer and grouping-by-applicant approaches. The grouping-by-referrer approach to define the job level (as used in the Firm 2 data) should be most precise for the lower job levels, and most noisy for higher job levels. This expectation comes from the likelihood that referrers at the lowest job level rarely refer applications for jobs at higher levels. Conversely, the most noisy parameter calculations would be for the higher job levels as referrers at the highest job level could refer applicants for all levels in the firm. What would the noisier parameter calculations at the highest level mean for our study? It would mean that the calculations for the highest job levels would be biased toward the firm-wide outcome rather than the job-level-specific outcome. And what would this bias mean for our results? This bias would mean that our observed pattern of a declining percentage female from network recruitment is conservative, and the true pattern would show a steeper negative slope. This steeper negative slope would be the only way we could observe our results in the context of mean-biased values for the higher job levels. That is, consider the lowest job-level calculation fixed (if it is the most precise), and that the highest job-level calculation has been pushed toward the firm mean through the assumptions underlying our calculations. Because the observed slope is negative, the only way the higher job-level values could have been pushed toward the firm mean would be if their true values were even lower than the current calculations—that is, a more steeply negative slope. This grouping-by-referrer was the case for Firm 2. If this method was also used for Firm 1, the pair of results would be similarly conservative.
Conversely, if Firm 1 used a grouping-by-applicant approach, then the highest job-level calculations would be the most precise because referral applicants to the highest job levels would be likely to receive those referrals only from others in the highest job levels, and least likely from those at lower job level). The lowest job-level calculations would be pushed toward the overall firm mean because the applicants at the lowest levels could have been referred by referrers at any level. In this case, the highest job-level calculations could be considered fixed, and the calculations of the lowest job levels would have been pushed toward the firm mean. Because our negative sloping pattern for Firm 1 has the highest job levels yielding the lowest percentage female equilibrium levels, the only way the lowest job levels could be at their calculated levels and biased toward the firm mean would be if the actual equilibrium for the lowest job levels were higher than what was calculated. Again, this would mean that the true slope would be more negative than the calculated trend for Firm 1.
We know the assumption underlying the parameter calculations for Firm 2. As we argue, we have reason to believe that the observed negative slopes are conservative estimates. This conclusion, plus the fact that we observe a similar negative slope with our data from Firm 1 both lend strength to our conclusion that network recruitment yields a glass ceiling pattern.
Theoretical and Practical Implications
The two goals were to bring empirical evidence to the prior assertion-based arguments regarding a possible role of network processes in contributing to a glass ceiling effect, and to demonstrate how existing organizational HR data resources can be leveraged to understand and manage the segregating effects of network recruitment. These two goals map well onto the theoretical and practical implications of this article.
In theoretical terms, we provide empirical evidence that network recruitment can contribute to the glass ceiling. But the nature of this evidence also challenges and changes the conventional wisdom explaining why network recruitment contributes to the glass ceiling. Network recruitment does not contribute to the glass ceiling simply because of homophily and the old boys’ network. Instead, network recruitment contributes to the glass ceiling because of gendered differences in the rates of who refers, and gendered differences in the rates in which referrers refer their same-sex contacts (on these dynamics, see Rubineau and Fernandez 2015). In the two firms we examined, the lowest-level jobs had the highest gender differences in rates of referring—both heavily favoring women engaging in referring. These differences contributed greatly to the strong integrating effects of network recruitment for the lowest-level jobs. These system-based insights into the segregating effects of network recruitment also have important practical implications for managing these effects.
In practical terms, we show that the HR data commonly collected by large firms in administering their recruitment efforts and referral bonus programs is enough to reveal the segregating effects of network recruitment not only for the firm overall, but even for narrower subsets of jobs within the firm. We show that the data need not be perfect or complete to be able to make these calculations. In the absence of detailed data, reasonable approximations along with sensitivity analyses can reveal whether the results would be sensitive to the more detailed data. Thus, firms seeking to evaluate the effects of their network recruitment practices on their goals for diversity can readily do so.
These methods can even help leverage network recruitment as a tool for improving and targeting diversity outcomes. For example, the current finding is that network recruitment acts as an integrating force, but more strongly for lower-level jobs. Without intervening in the network recruitment processes for higher-level jobs, the expectation may be a gradual gender integration of the firm. This integration, however, would be more substantial for lower-level jobs and would be unlikely to ameliorate the gendered barriers apparent for higher-level jobs. Moreover, our analyses suggest that policies designed to encourage the underrepresented group to participate in referring are more likely to harness the integrating powers of network recruitment than are policies that seek to specifically target the underrepresented group.
We conclude with confidence that the pattern of network recruitment does contribute to a glass ceiling effect in these two firms. As noted, our empirical setting is not representative of any larger set of firms, so our conclusion does not imply such a pattern in other firms. The firms selected were a kind of convenience sample of firms collecting and willing to share their recruitment and referral data. They were not identified based on their being more or less likely to exhibit a glass ceiling phenomenon or to have such a phenomenon associated with their referring practices. Still, given that ours is the first study to empirically assess whether network recruitment contributes to the glass ceiling, we find it striking that we find strong and consistent results in the first two settings we examine. This result could be due to chance. It could also suggest that network recruitment commonly contributes to the glass ceiling. Determining which of these two explanations is more correct requires replicating this study in more organizational settings.
Regardless of whether these results generalize to other settings, the cooperating firms find clear value in assessing whether and how network recruitment dynamics contribute to gender disparities across levels of their organizations. As the firms craft their policies on these issues, that these analyses correspond to their circumstances is an appealing rather than a limiting feature. In their cases, one size needn’t fit all when developing their policy prescriptions.
These analyses isolate and clarify the contribution of recruitment network dynamics affecting the glass ceiling. However, other biases and composition-altering mechanisms likely operate in real-world recruitment processes. Although the calculated equilibriums for network recruitment effects represent the segregating effects of network recruitment in isolation, we cannot claim that these affects are a complete explanation for why we so often find glass ceiling patterns in organizations. We can, however, offer some general guidance about the relative importance of such network recruitment factors.
Although other forces may affect a given firm’s recruitment process, we can say that, for firms that do not rely heavily on network recruitment, the segregating effects of network recruitment are expected to be very weak. At the same time, for firms that do rely heavily on network recruitment, the segregating effects of this mode of recruitment are expected to be stronger. To build intuition about the relative magnitude of these network recruitment factors, we have developed a way of representing the size of these effects in terms of one of those other biasing factors, that is, sex biases in screening (see Rubineau and Fernandez 2013). The calculated equilibriums we present for network recruitment in these two firms provides a measure of the direction and magnitude of the composition-altering effects of network recruitment—the segregating or desegregating push or pull of network recruitment. The magnitude of the network factors we uncovered in these two settings are equivalent to an overall preference for female applicants that resembles a 56:44 or 57:43 chance (the equivalent of odds of 1.28 to 1.30) for hiring women relative to men, rather than a gender-neutral 50:50 chance.
In conclusion, although other factors may be at work at producing the glass ceiling, at least in these settings, network recruitment dynamics are a meaningful part of their stories. Revealing these network recruitment dynamics can be an important step toward managing these processes to support and achieve firms’ diversity goals. These insights are made possible only by partnering with these firms to gain access to these commonly available but rarely analyzed administrative HR data.
FOOTNOTES
↵1. In the United States, firms of fifty or more employees are legally required to maintain such records.
↵2. In theory, it is possible for the data for these disparate processes to be contained in a single relational database. In practice, however, we have yet to encounter examples of such integrated end-to-end HR databases.
↵3. See “Determine the gender of a first name,” https://genderize.io/#overview (accessed October 15, 2018).
↵4. For the input composition, we use the percent female of nonreferral applicants. In the hypothetical case where all other composition-altering mechanisms were removed, the equilibrium gender composition of the firm would necessarily be the composition of its inputs—nonreferral job applicants.
↵5. These integrating processes are from the firms’ behaviors affecting the flow of candidates into the firm, and not from any screening preference. Indeed, as discussed in Rubineau and Fernandez (2013, 2015), this is one of the appealing features of using network recruitment as an integrating lever. In an era when firms are shy about implementing affirmative action as hiring preferences, “pipeline stoking” processes are particularly attractive.
↵6. We have come across two examples where companies have sought to encourage this second lever in their diversity efforts. Pinterest and Intel both have instituted higher referral bonuses whenever people refer a woman or a minority than when they refer a white male. Interestingly, we had judged the use of this kind of targeted referring as ethically and legally questionable (Rubineau and Fernandez 2015).
- © 2019 Russell Sage Foundation. Fernandez, Roberto M., and Brian Rubineau. 2019. “Network Recruitment and the Glass Ceiling: Evidence from Two Firms.” RSF: The Russell Sage Foundation Journal of the Social Sciences 5(3): 88–102. DOI: 10.7758/RSF.2019.5.3.05. Direct correspondence to: Roberto M. Fernandez at robertof{at}mit.edu, MIT Sloan School of Management, Massachusetts Institute of Technology, Room E62–387, 100 Main St., Cambridge, MA 02142.
Open Access Policy: RSF: The Russell Sage Foundation Journal of the Social Sciences is an open access journal. This article is published under a Creative Commons Attribution-NonCommercial-NoDerivs 3.0 Unported License.